Foundation Prize winner Aron K. Barbey’s groundbreaking research reveals connection between neural architecture and intelligence
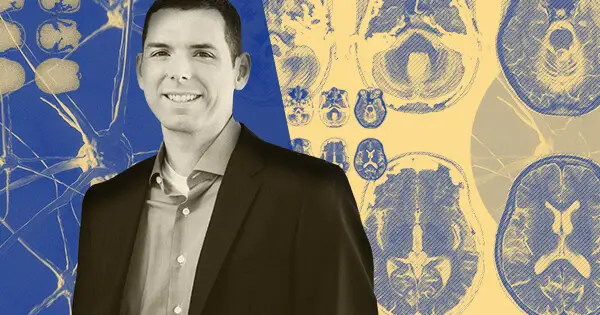
Like the brain, studying human intelligence can be curious, particularly when it comes to correlating quantitative measurements with specific biological factors.
That is what makes so impressive the research of Professor Aron K. Barbey, director of the Center for Brain Plasticity at the University of Illinois Urbana-Champaign and winner of the 2019 Mensa Foundation Prize. His work with functional magnetic resonance imaging to map brain lesions and measure their effects on raw intelligence has significantly advanced the neuroscience of brain connectivity. Mensa Bulletin editor Chip Taulbee spoke with Dr. Barbey about he and his fellow researchers’ breakthrough invesitgations.
Mensa Bulletin: Your research at the Beckman Institute for Advanced Science and Technology has been instrumental to understanding the neural foundations of human intelligence. Can you tell us about the inception of this work and why a neuroscientific perspective is important for understanding human intelligence?
Professor Aron K. Barbey: Research in the psychological and brain sciences has long sought to understand the nature of human intelligence, examining the stunning breadth and diversity of intellectual abilities and the remarkable neural mechanisms from which they emerge. The foundations of modern research in this effort were established in the early 20th century by Charles Spearman, who developed the correlation method and applied this technique to examine academic achievement. Spearman discovered that correlations in performance reflected characteristics of each discipline — writing, for example, that “English and French agree with one another in having a higher correlation with Classics than with Mathematics.”
Beyond identifying the contribution of specific mental abilities, Spearman observed that the correlations among academic disciplines were always positive. This finding, which is now well established and called the positive manifold, provided evidence that all cognitive tests measure something in common. Spearman referred to this commonality as the general factor, g, which represents the component of individual differences variance that is common across all tests of mental ability. These early findings motivated Spearman’s two-factor model, which held that performance on tests of mental ability jointly reflect a specific factor, s, that is unique to each test, and a general factor, g, that is common across all tests.
Contemporary research has further elaborated Spearman’s model to include an intermediate level of broad abilities that account for the variance that is shared across similar domains of cognitive ability. For example, the well-established Cattell—Horn—Carroll theory — developed by University of Illinois Professor Raymond Cattell and his colleagues — distinguishes between performance on tests of prior knowledge and experience, referred to as crystallized intelligence, from those that require adaptive reasoning in novel situations, called fluid intelligence. Taken together, the specific, broad, and general factors of intelligence account for the hierarchical pattern of correlations that are observed among tests of mental ability.
Researchers at the University of Illinois studied survivors of brain injury to map the architecture of intelligence, and whether damage to specific regions of the brain can be mapped to specific post-injury impairments, including measurements of working memory, and visual and spacial reasoning. The Science Coalition
Spearman’s discoveries ushered in a new era of research on human intelligence and uncovered fundamental mysteries about the nature and origins of g that stand as one of the most significant and enduring challenges for modern research in the psychological and brain sciences. Despite the fact that g represents the largest component of the common factor variance, its psychological foundations have remained largely invisible and beyond the reach of further scientific examination.
The enigmatic nature of general intelligence arises from the fact that g is not a measure of specific knowledge, skills, or strategies for problem-solving. These aspects of task performance are simply a vehicle for the measurement of g. The general factor instead accounts for individual differences in information processing at a global level. Thus, we cannot understand the causal underpinnings of g by appealing to specific cognitive processes or by directly examining the psychological tests from which the general factor is derived.
Research on the nature and origins of g must therefore extend beyond psychology to examine the neurobiological mechanisms that shape individual differences in cognitive ability. The interdisciplinary field of network neuroscience enables the formal measurement and modeling of the interactions among network elements, providing a powerful new lens for examining the emergence of global network phenomena.
As founding Director of the Center for Brain Plasticity, can you tell us how brain plasticity contributes to general intelligence?
The Center for Brain Plasticity reflects a collaborative effort, with major contributions from my colleagues Neal Cohen and Jeff Moore at Illinois and the remarkable community of world-class faculty, students, and staff at the Beckman Institute for Advanced Science and Technology.
The capacity for flexible, intelligent behavior is made possible by the brain’s remarkable capacity to reconfigure itself — to continually update prior knowledge on the basis of new information and to actively generate internal predictions that guide adaptive behavior and decision making. Contemporary research conceives of the brain as a dynamic and active inference generator that anticipates incoming sensory inputs, forming hypotheses about that world that can be tested against sensory signals that arrive in the brain. Plasticity is therefore critical for the emergence of human intelligence — providing a powerful mechanism for updating prior beliefs, generating dynamic predictions about the world, and adapting in response to ongoing changes in the environment.
We have developed a network neuroscience theory of general intelligence that is based on these ideas. According to the theory, general intelligence reflects individual differences in the efficiency and flexibility of brain networks. The human brain is designed for efficiency — to minimize the cost of information processing while maximizing the capacity for growth and adaptation. Accumulating evidence indicates that general intelligence is associated with global efficiency, the capacity to integrate information across the brain as a whole.
Flexibility is afforded by brain plasticity. Network flexibility is shaped by the structural and functional organization of the brain, which may facilitate or constrain the transition of a network from one state to another. For example, transitioning to an easy-to-reach state requires a short, direct path, whereas transitioning to a difficult-to-reach state requires a long, winding path. The network neuroscience theory proposes that crystallized intelligence engages highly accessible representations of prior knowledge and experience and relies on easy-to-reach network states. In contrast, fluid intelligence reflects the capacity to solve novel problems and to demonstrate adaptive, flexible behavior. Fluid intelligence therefore engages networks that can transition to difficult-to-reach, highly flexible states. Thus, rather than attribute intelligence to a fixed set of brain regions or networks, this perspective is based on the dynamic reorganization of brain networks and proposes that intelligence is grounded in brain plasticity.
Our goal is to advance research and theory on the neurobiology of human intelligence by incorporating evidence from network neuroscience on the global topology and dynamics of the human brain.
Can you tell us about the major advances in the field that have occurred as a consequence of your research?
Our research contributes to a wealth of evidence investigating the nature and mechanisms of human intelligence. We are editing a forthcoming Cambridge Handbook on Intelligence and Cognitive Neuroscience — with my colleagues Sherif Karama at McGill and Richard Haier at U.C. Irvine — that will provide an excellent review of modern research and theory on the neurobiology of human intelligence. I am also editing a special issue in Trends in Neuroscience and Education with my colleague Michael Posner at the University Oregon on the “Cognitive Neuroscience of Human Intelligence.”
Early research in this field began by adopting a localizationist approach, attempting to uncover specific brain regions that contribute to individual differences in cognitive ability. Over the years, there have been important proposals about the role of specific brain regions, primarily within the prefrontal cortex, that facilitate general intelligence. Our early work, for example, found that damage to the prefrontal cortex, specifically within a region called the dorsolateral prefrontal cortex, selectively impaired general intelligence. However, it became clear that although there were indeed specific regions that were important for general intelligence, these regions did not operate in isolation but instead participated in broadly distributed networks. A major advance in the field was therefore to go beyond the specification of individual regions to assess brain networks and the communication pathways that are important for general intelligence.
Over the years, then, scientists discovered specific brain networks that account for individual differences in cognitive ability. And those networks were comprised not only of regions within the prefrontal cortex but also regions within other areas of the brain. One of the primary networks that our group has studied comprises regions within the frontal and parietal cortex, a network called the frontoparietal network. We found that selective damage to this network impairs general intelligence and this work helped motivate network-based theories of general intelligence. A number of other networks have been recently identified, but the main transition that occurred in the field was to move away from focusing on isolated brain regions to examine the role of distributed brain networks in general intelligence. More recently, the field has increasingly adopted theories and methods from the field of network neuroscience — in an effort to understand the global topology and dynamics of the human brain and their roles in general intelligence.
A second major development was to broaden the scope of research in the field to include emotional intelligence and social problem solving. A longstanding question concerns whether general intelligence primarily reflects logical thought and reason or whether it also relies on emotions and feelings. We conducted one of the first neuroscience studies of emotional intelligence, the capacity to perceive and understand our own emotions and those of others, and to use this information to guide thought and behavior. We found that emotional intelligence engaged brain networks that are also implicated in general intelligence, motivating an integrative approach to understanding the role of executive, social, and emotional processes in general intelligence.
Problem-solving, decision making, memory, and other cognitive processes involve the joint activity of more than one person. The other is outsourcing: The knowledge people use often sits in the head of someone else.
A third, more recent development, recognizes that knowledge is distributed within the community and that general intelligence is therefore a social entity. This work is conducted with my colleagues Steven Sloman at Brown and Richard Patterson at Emory. According to the community of knowledge hypothesis, there are two ways in which the cognitive abilities of the individual depend on others. One is collaboration: Problem-solving, decision making, memory, and other cognitive processes involve the joint activity of more than one person. The other is outsourcing: The knowledge people use often sits in the head of someone else. For instance, our explanations often appeal to causal models that sit in the heads of scientists and engineers. More generally, people’s sense of understanding, reasoning, decision making, and use of words and concepts are often outsourced to others, and often we don’t know who we are outsourcing to. For instance, when we say “they landed on the moon,” most of us have little idea who they refers to.
Thus, the community of knowledge hypothesis implies that there is no static neurobiology underlying general intelligence, specifically the cognitive abilities that support the emergent thinking that groups and communities engage in. And that’s most thinking. Greater understanding of how people collaborate would help reveal how neural processing makes use of group dynamics and affiliation, and it would support a more realistic model of mental activity that acknowledges individual limitations.
Cognitive neuroscience is already making marked progress on these questions. Greater understanding of how people outsource would help reveal the actual nature and limits of neural representation, and shed light on how people organize information by revealing how they believe it is distributed in the community and the world. And greater appreciation of the emergent nature of knowledge in society would help us recognize the limits of cognitive neuroscience, that the study of the brain alone cannot reveal the representations responsible for activities that involve multiple people. It is hard to overestimate how much of what we do depends on other people.
What are the primary questions that drive your current research on the neurobiology of human intelligence?
Our research at the Decision Neuroscience Laboratory is made possible by a remarkably talented team of research scientists, postdoctoral fellows, graduate students, and staff. Our work, one, promotes an interdisciplinary approach to understanding the nature and mechanisms of human intelligence, calling for a synthesis of research across the psychological, brain, and computational sciences; two, sets the stage for major advances in the scientific effort to enhance cognitive performance, investigating the efficacy of integrative skill training, mindfulness meditation, non-invasive brain stimulation, diet and nutrition, and physical activity and aerobic fitness; and, three, demonstrates how the scientific effort to improve the mind is fundamentally changing our understanding of human intelligence — supporting new perspectives about its dynamic and adaptive nature and motivating new insights about how intelligence emerges through evolution and development, is cultivated through learning and prior experience, and is altered through psychiatric illness and neurological disease.
Our aim is to conduct research that has broad, transformative impact — guiding public policy recommendations about the nature of human intelligence in education, business, artificial intelligence, and medicine.
What implications do you believe research on human intelligence has for the future of artificial intelligence and the design of new technologies to promote machine intelligence?
I am currently editing a special issue in the journal Intelligence that addresses this topic, entitled “Advancing the Science of Human and Machine Intelligence: Forging Connections between Psychology, Neuroscience, and Engineering.”
Modern advances in computer science and engineering demonstrate the potential of new technological tools and statistical methods to assess intelligent behavior and to imbue machines with new capabilities to positively impact human performance and society. Recent insights have been made, for example, by my colleagues in Electrical and Computer Engineering at Illinois, Lav Varshney and Naresh Shanbhag.
There are a wealth of new technologies and statistical methods that have been developed to drive innovation in human-centered machine intelligence. The aim is to draw upon discoveries in human neuroscience about the information processing architecture of general intelligence and to use these insights to guide theory and applications in computer science and engineering.
Life scientists, computer scientists, social scientists, and engineers are currently working together to inspire the next generation of theories and tools to advance the science of both human and machine intelligence. The implications of these discoveries for human-computer interaction, performance monitoring and optimization, and human mental life and society will be transformative, progressively changing science fiction — the world of Frank Herbert or the vision of Ridley Scott — into modern reality.
Comments (0)